SEMI-AUTOMATED OBJECT DETECTION WORKLOWS USING UAV IMAGERY
Send your question(s) for discussion within the Q&A!
In order to provide meaningful discussions in the Q&A with Samuel Oswald, you can send us your questions & we will cover them during the Q&A on June 17th, 14:00 CEST.
Simply send them by mail: ippn@plant-phenotyping.org
Click here to join live Q&A session on 17 June, 14:00 CEST
Abstract
In plant phenotyping, researchers are often interested in measuring the timing and distribution of plant emergence, as well as the density of organs on a mature plant. By using high-resolution UAV imagery and modern machine learning model architectures, it is possible to predict the density of these objects on a much greater scale with less manual work required. Difficulties remain however, in the scaling up of these models, with most showing accurate results only when applied to a restricted target region.This is due to differences in species morphology, however is also caused by differences in the images themselves, onset by disparate collection plans, as well as variable image quality.
To overcome this, we have developed an end-to-end image processing platform (MAPEO), which allows researchers to collect and process UAV flights at scale, outputting several products and providing standardised imagery outputs which can be compared spatially and temporally. This workflow extends from the initial flight parametization, to collection and in-field quality assessment, through to online processing and publishing.
Outputs are standardised and with attached metadata can be incorporated into additional machine learning pipelines. This allows increasingly high amounts of data to be leveraged in order to train increasingly robust models, covering various field set-ups in terms of environmental setting and the species morphologies present. Additionally, fine-tuning is optimized towards target phenotyping problems. Overall, researchers are able to spend less labour and computational time but still retrieve more reliable and accurate results as compared to ad hoc object detection modelling. Test results show that this workflow is able to produce better results when adapting model architectures to phenotyping use cases as compared to out-of-the-box architectures. Additionally, test cases for wheat-ear detection has shown models trained from datasets which were collected under similar flight conditions can be applicable to each other, increasingly so as more variability in wheat morphologies and environmental conditions is included.
Speaker Information
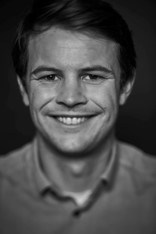
Short Bio:
Samuel Oswald is a data scientist working at VITO Remote Sensing within the MAPEO team. MAPEO aims to empower researchers with access to drone data and the insights it generates. Samuel primarily works on the implementation and improvement of advanced machine learning algorithms, allowing users to leverage the power of diverse data sources and optimized machine learning architectures to detect several interesting features on their trial fields. He is also interested in co-operating on new research ideas or how helping researchers apply machine learning to their own projects.